PhD Proposal: Kamal Aryal
Location
Physics : 401
PhD Proposal: Kamal Aryal – Online Event
Date & Time
April 21, 2022, 2:00 pm – 4:00 pm
Description
ADVISOR: Dr. Pengwang Zhai
TITLE: Efficient retrieval of aerosol and ocean water properties from multiangle polarimetric measurements over coastal waters using Neural network
ABSTRACT: Ocean color remote sensing using multangular polarimeters (MAPs) plays a key role to advance ocean ecosystem and aerosol studies as it provides rich information on microphysical properties of aerosols and hydrosols. Several joint retrieval algorithms have been developed to retrieve inherent optical properties (IOPs) of ocean waters and aerosols simultaneously by fitting the MAPs measurements. The applicability of the developed joint retrieval algorithm based on the MAP measurements depends on the representativeness of the bio-optical models on the IOPs of ocean waters. For open ocean waters IOPs are parameterized by only chlorophyll-a concentration ([Chla]) as they covary. However, over coastal waters, where IOP’s do not tightly co-vary with [Chla] more sophisticated bio-optical models including absorption and scattering by marine particulates and Colored Dissolved Organic Material (CDOM) are needed in order to retrieve aerosol and ocean properties.
Full exploitation of the information content in MAP measurements using these retrieval algorithms over coastal waters faces several challenges. Particularly a large number of retrieval parameters in joint retrieval algorithms lead to increased iterative calls to the forward model, which is computationally expensive and difficult to operationally process satellite dataset on a global scale. This problem can be tackled by exploring the possibility of reducing free retrieval parameters while maintaining the retrieval accuracy and by developing computationally efficient retrieval algorithms suitable for operational processing of the large data volumes acquired by the MAP imagers.
In this work, we propose to develop a joint retrieval algorithm for coastal waters using Neural Network (NN) to replace the forward model calculation which will have smaller parameter space to represent both aerosol and water IOP’s. The bio-optical model to represent water IOP’s will have four parameters which are found to be sufficient in a recent study. Atmospheric aerosols will be represented as the mixture of different volume fractions of known components with fixed size distribution and refractive index spectra with six retrieval parameters including relative humidity and fine mode fraction. There will be a total of 11 retrieval parameters. Neural network forward model will be trained, tested, and validated using a large volume of synthetic datasets simulated using the radiative transfer model based on the successive order of scattering method (RTSOS). Thus, the developed retrieval algorithm will be used in simultaneous retrieval of aerosol and water IOP’s using HARP instruments.
Another goal of this work focuses on understanding the vertical distribution of downwelling irradiance in the visible spectrum inside both open ocean and coastal waters. For this purpose, we propose two IPAR (Instantaneous photosynthetically available radiation) prediction models based on a neural network (NN) approach, with different networks for open ocean and coastal waters. The performance of the NN models is better than presently available semi analytical models in both waters with significant superiority in coastal waters.
Kamal Aryal's proposal defense
Hosted by Pengwang Zhai
https://umbc.webex.com/umbc/j.php?MTID=mc1a6d8779b8f0f614e0ff076660e7704
Thursday, Apr 21, 2022 2:00 pm | 2 hours | (UTC-04:00) Eastern Time (US & Canada)
Meeting number: 2622 693 9892
Password: JkbNHXnN564
Join by video system
Dial 26226939892@umbc.webex.com
You can also dial 173.243.2.68 and enter your meeting number.
Join by phone
+1-202-860-2110 United States Toll (Washington D.C.)
Access code: 262 269 39892
TITLE: Efficient retrieval of aerosol and ocean water properties from multiangle polarimetric measurements over coastal waters using Neural network
ABSTRACT: Ocean color remote sensing using multangular polarimeters (MAPs) plays a key role to advance ocean ecosystem and aerosol studies as it provides rich information on microphysical properties of aerosols and hydrosols. Several joint retrieval algorithms have been developed to retrieve inherent optical properties (IOPs) of ocean waters and aerosols simultaneously by fitting the MAPs measurements. The applicability of the developed joint retrieval algorithm based on the MAP measurements depends on the representativeness of the bio-optical models on the IOPs of ocean waters. For open ocean waters IOPs are parameterized by only chlorophyll-a concentration ([Chla]) as they covary. However, over coastal waters, where IOP’s do not tightly co-vary with [Chla] more sophisticated bio-optical models including absorption and scattering by marine particulates and Colored Dissolved Organic Material (CDOM) are needed in order to retrieve aerosol and ocean properties.
Full exploitation of the information content in MAP measurements using these retrieval algorithms over coastal waters faces several challenges. Particularly a large number of retrieval parameters in joint retrieval algorithms lead to increased iterative calls to the forward model, which is computationally expensive and difficult to operationally process satellite dataset on a global scale. This problem can be tackled by exploring the possibility of reducing free retrieval parameters while maintaining the retrieval accuracy and by developing computationally efficient retrieval algorithms suitable for operational processing of the large data volumes acquired by the MAP imagers.
In this work, we propose to develop a joint retrieval algorithm for coastal waters using Neural Network (NN) to replace the forward model calculation which will have smaller parameter space to represent both aerosol and water IOP’s. The bio-optical model to represent water IOP’s will have four parameters which are found to be sufficient in a recent study. Atmospheric aerosols will be represented as the mixture of different volume fractions of known components with fixed size distribution and refractive index spectra with six retrieval parameters including relative humidity and fine mode fraction. There will be a total of 11 retrieval parameters. Neural network forward model will be trained, tested, and validated using a large volume of synthetic datasets simulated using the radiative transfer model based on the successive order of scattering method (RTSOS). Thus, the developed retrieval algorithm will be used in simultaneous retrieval of aerosol and water IOP’s using HARP instruments.
Another goal of this work focuses on understanding the vertical distribution of downwelling irradiance in the visible spectrum inside both open ocean and coastal waters. For this purpose, we propose two IPAR (Instantaneous photosynthetically available radiation) prediction models based on a neural network (NN) approach, with different networks for open ocean and coastal waters. The performance of the NN models is better than presently available semi analytical models in both waters with significant superiority in coastal waters.
Kamal Aryal's proposal defense
Hosted by Pengwang Zhai
https://umbc.webex.com/umbc/j.php?MTID=mc1a6d8779b8f0f614e0ff076660e7704
Thursday, Apr 21, 2022 2:00 pm | 2 hours | (UTC-04:00) Eastern Time (US & Canada)
Meeting number: 2622 693 9892
Password: JkbNHXnN564
Join by video system
Dial 26226939892@umbc.webex.com
You can also dial 173.243.2.68 and enter your meeting number.
Join by phone
+1-202-860-2110 United States Toll (Washington D.C.)
Access code: 262 269 39892
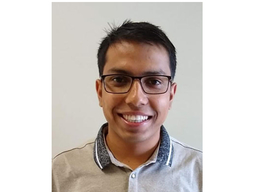