PhD Proposal: Akram Ibrahim
Location
Physics : 401
Date & Time
April 21, 2022, 11:00 am – 12:30 pm
Description
ADVISOR: Dr. Can Ataca
TITLE: Understanding growth of low-dimensional materials using multiscale modeling and machine learning
ABSTRACT: Low-dimensional materials have emerged as an important class of materials for novel applications in optoelectronics, spintronics, energy storage and conversion technologies. In order to achieve mass production of low-dimensional materials, we need to synthesize these materials at large scales and high quality. However, most of the successful experimental methods still suffer from the existence of uncontrolled defects occurring during the growth process. This is due to the complexity of the mechanisms associated with crystal growth that take place on timescales that cannot be observed with the current experimental technologies. Over the last decade, a remarkable progress has been made in the use of computational methods for understanding the growth mechanisms and predicting the properties of low-dimensional materials. Computational methods can save a lot of the cost of experimental work in terms of time and resources. However, the modeling of the crystal growth process requires the computational model to cover a wide range of length and time scales simultaneously, ranging from a nanometer to a few millimeters, and from femtoseconds to a few seconds. Because of the direct relationship between cost and required accuracy, conventional modeling is ill suited for this task and multiscale modeling is the appropriate choice due to its compromise between cost and accuracy. Current multiscale modelling techniques depend on parametrization to transfer the information from the first-principles computational methods to the coarse-grained models at large scales. Nevertheless, parametrized models often suffer from their limited accuracies that can deviate significantly from the experimental reality. Here, we depend on the new emerging field of machine learning potentials to bridge the knowledge from accurate but scale-limited first-principles methods such as density functional theory and quantum Monte Carlo to the large-scale simulation methods such as molecular dynamics and kinetic Monte Carlo. This approach can unlock the riches of the detailed growth processes of low-dimensional materials.
TITLE: Understanding growth of low-dimensional materials using multiscale modeling and machine learning
ABSTRACT: Low-dimensional materials have emerged as an important class of materials for novel applications in optoelectronics, spintronics, energy storage and conversion technologies. In order to achieve mass production of low-dimensional materials, we need to synthesize these materials at large scales and high quality. However, most of the successful experimental methods still suffer from the existence of uncontrolled defects occurring during the growth process. This is due to the complexity of the mechanisms associated with crystal growth that take place on timescales that cannot be observed with the current experimental technologies. Over the last decade, a remarkable progress has been made in the use of computational methods for understanding the growth mechanisms and predicting the properties of low-dimensional materials. Computational methods can save a lot of the cost of experimental work in terms of time and resources. However, the modeling of the crystal growth process requires the computational model to cover a wide range of length and time scales simultaneously, ranging from a nanometer to a few millimeters, and from femtoseconds to a few seconds. Because of the direct relationship between cost and required accuracy, conventional modeling is ill suited for this task and multiscale modeling is the appropriate choice due to its compromise between cost and accuracy. Current multiscale modelling techniques depend on parametrization to transfer the information from the first-principles computational methods to the coarse-grained models at large scales. Nevertheless, parametrized models often suffer from their limited accuracies that can deviate significantly from the experimental reality. Here, we depend on the new emerging field of machine learning potentials to bridge the knowledge from accurate but scale-limited first-principles methods such as density functional theory and quantum Monte Carlo to the large-scale simulation methods such as molecular dynamics and kinetic Monte Carlo. This approach can unlock the riches of the detailed growth processes of low-dimensional materials.
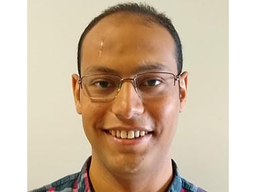