MS Thesis Defense: Prajwal Panda
Location
Physics : 401
Date & Time
November 21, 2023, 1:00 pm – 3:00 pm
Description
ADVISOR: Dr. Mark Henriksen
TITLE: New Methods to Characterize the Substructure in the Abell 2146 Cluster of Galaxies
ABSTRACT: Unraveling the complexities of galaxy clusters is a pivotal endeavor in astrophysics. In this thesis, we employ state-of-the-art unsupervised learning techniques, specifically Gaussian Mixture Model (GMM) and K-means clustering, to explore the intricate structures of the Abell 2146 galaxy cluster. Using the spatial coordinates of Right Ascension (RA) and Declination (Dec), and velocity as the third dimension, we endeavor to determine the optimal number of sub-structures within this celestial cluster.
Strikingly, our analysis reveals that K-means, among the two clustering techniques, offers superior performance in unraveling the cluster’s inherent structures. We use two powerful methods, the silhouette and elbow methods, to effectively optimize the number of sub structures.
With the optimal number of clusters now ascertained, we extend our investigation to apply the spherical infall model. This phase focuses on the kinematics of the sub-structures found within the cluster, and their relationship to the density of the cluster. We test out findings with two of the most common density models, Ωm = 0.3 and Ωm = 0.04 , where Ωm = ρ/ρc, accommodating both dark and luminous matter contributions. Through our investigation, we constrain the mass density dynamics of the Abell 2146 galaxy cluster.
This interdisciplinary approach harmonizes cutting-edge machine learning with astrophysical modeling, shedding new light on the nature of galaxy clusters. By combining these methodologies, our research not only advances our understanding of cosmic structures but also elucidates the complex interactions that define the structure formation in the Universe.
TITLE: New Methods to Characterize the Substructure in the Abell 2146 Cluster of Galaxies
ABSTRACT: Unraveling the complexities of galaxy clusters is a pivotal endeavor in astrophysics. In this thesis, we employ state-of-the-art unsupervised learning techniques, specifically Gaussian Mixture Model (GMM) and K-means clustering, to explore the intricate structures of the Abell 2146 galaxy cluster. Using the spatial coordinates of Right Ascension (RA) and Declination (Dec), and velocity as the third dimension, we endeavor to determine the optimal number of sub-structures within this celestial cluster.
Strikingly, our analysis reveals that K-means, among the two clustering techniques, offers superior performance in unraveling the cluster’s inherent structures. We use two powerful methods, the silhouette and elbow methods, to effectively optimize the number of sub structures.
With the optimal number of clusters now ascertained, we extend our investigation to apply the spherical infall model. This phase focuses on the kinematics of the sub-structures found within the cluster, and their relationship to the density of the cluster. We test out findings with two of the most common density models, Ωm = 0.3 and Ωm = 0.04 , where Ωm = ρ/ρc, accommodating both dark and luminous matter contributions. Through our investigation, we constrain the mass density dynamics of the Abell 2146 galaxy cluster.
This interdisciplinary approach harmonizes cutting-edge machine learning with astrophysical modeling, shedding new light on the nature of galaxy clusters. By combining these methodologies, our research not only advances our understanding of cosmic structures but also elucidates the complex interactions that define the structure formation in the Universe.
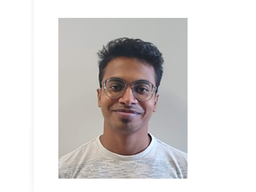