Seminar: Dr. Maria Janeth Molina | UMD
In-Person PHYS 401
Location
Physics : 401
Date & Time
November 13, 2024, 11:00 am – 12:00 pm
Description
TITLE: "Machine learning for Earth system prediction and predictability”
ABSTRACT: Machine learning can be used for Earth system prediction, or to study the uppermost limit of prediction skill theoretically achievable given the system's initial state or other factors, otherwise known as predictability. In traditional numerical weather prediction frameworks, we solve the governing partial differential equations starting from an initial state. This initialized prediction framework usually involves three stages: 1) generating the initial conditions of the Earth system, 2) running the mathematical representation of the system on a computer forward in time, and 3) analyzing the output and converting it into a format that is useful for end users. Machine learning can be used to improve each of these individual stages, or to circumvent the three stage framework altogether, and examples of each will be given in this seminar. More time during the seminar will be dedicated to the challenges surrounding subseasonal prediction, which focuses on lead times of three to four weeks, and how we can use machine learning to both uncover potential biases in our initialized prediction systems and how we can bias-correct them.
ABSTRACT: Machine learning can be used for Earth system prediction, or to study the uppermost limit of prediction skill theoretically achievable given the system's initial state or other factors, otherwise known as predictability. In traditional numerical weather prediction frameworks, we solve the governing partial differential equations starting from an initial state. This initialized prediction framework usually involves three stages: 1) generating the initial conditions of the Earth system, 2) running the mathematical representation of the system on a computer forward in time, and 3) analyzing the output and converting it into a format that is useful for end users. Machine learning can be used to improve each of these individual stages, or to circumvent the three stage framework altogether, and examples of each will be given in this seminar. More time during the seminar will be dedicated to the challenges surrounding subseasonal prediction, which focuses on lead times of three to four weeks, and how we can use machine learning to both uncover potential biases in our initialized prediction systems and how we can bias-correct them.
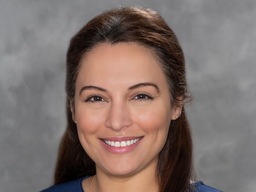